blog
How Predictive Quality Analytics Increased Battery Cell Manufacturing Yield By 16%
oct 8, 2019

Manufacturing battery cells for electric cars is challenging. That’s because production yield is typically low, the final quality validation requires a long test period, and the overall process is costly. This article explains how predictive quality analytics increased production yield by 16% for battery cells.
Elisa Smart Factory team conducted the project at eLab, which is the electromobility research center at the Aachen University, Germany.
What’s the quality challenge in battery cell manufacturing?
The manufacturing of Lithium-Ion battery cells for electric cars is, in principle, a straightforward process.
First, anode and cathode electrodes are produced in several different sub-processes from a mixture of different raw materials. Then, they are packaged into battery cells, filled with electrolyte, closed and sealed. Finally, the battery cells go through the end-of-line testing.
In reality, the process is much more complicated. One of the most challenging part of the process is the time it takes to verify the final quality of the produced battery cells. In fact, it can take up to three weeks to complete the end-of-line testing. And, it’s only after this point the manufacturer can determine if the final product is good enough for the battery pack production.
In case of non-sufficient quality, the manufacturer has to scrap the battery cell as hazardous waste. This is due to lack of cost-neutral recycling options. As a result, scarce, non-renewable and expensive raw materials such as lithium, cobalt, nickel sulfate, copper, aluminium, and graphite end up as waste.
The global average first-time-yield (FTY) for battery cells is as high as 15 percent. For this reason, battery cell manufacturing is costly and slow.
How to increase manufacturing quality of battery cells?
eLab (Electric Mobility Lab) at Aachen University, is working on the future of battery production. Also for them, quality issues as the primary challenge in the manufacturing process. In fact, it prohibits cost-efficient manufacturing and thus slows down the adoption of environmentally-friendly electric cars. On the quest for developing a more efficient manufacturing process, eLab partnered with Elisa Smart Factory data scientist team.
Why did we use predictive quality analytics?
Predictive quality analytics makes it possible to improve manufacturing quality, among other benefits. It’s the process of extracting useful insights from a manufacturing process by applying statistical algorithms and machine learning to determine patterns that can predict problems in advance. Thus, it was an appropriate tool to solve eLab’s quality challenge.
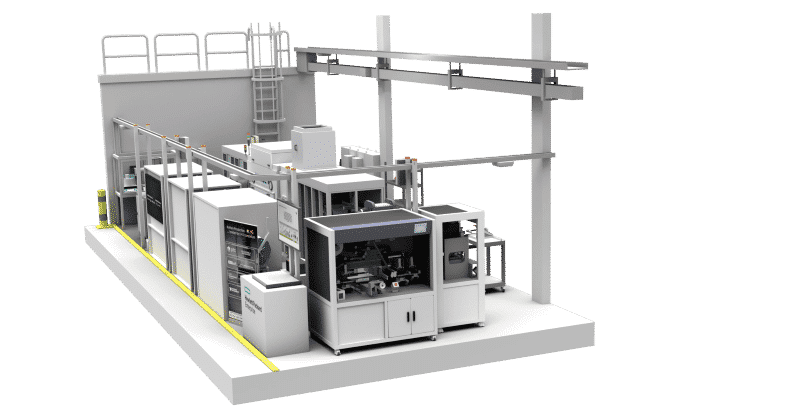
eLab Elisa Smart Factory 3D Digital Twin
How To Improve Resilience And Grow Your Business Sustainably
Unexpected disruptive events in manufacturing always interrupt normal production conditions and cause production loss. How resilient are your planning and manufacturing processes? Are you prepared to deal with the unexpected?
Take the quiz below to find out how your organisation stacks up in the face of crisis and get expert insights on how to make your manufacturing more resilient.